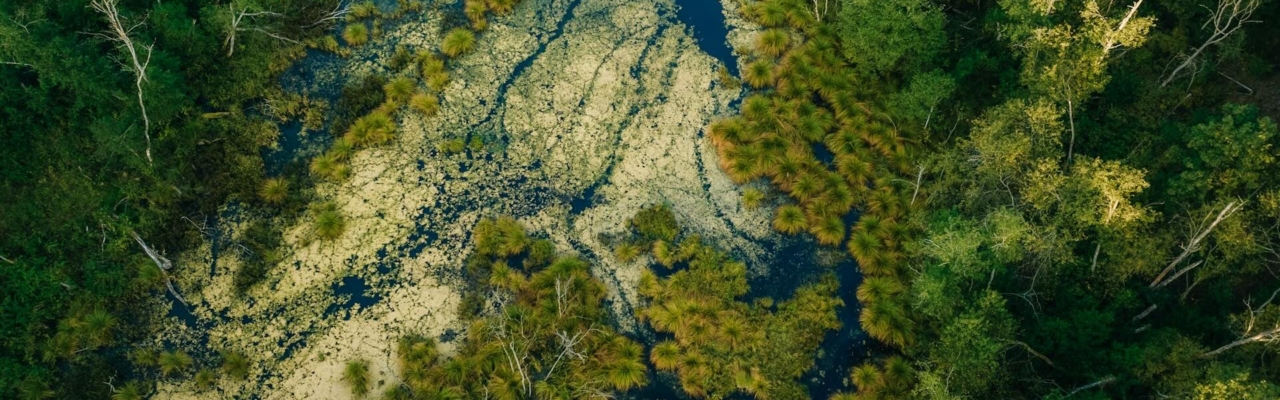
Machine learning is increasingly being used to study and protect nature. However, some of the drawbacks related to its use include environmental sustainability concerns which lead to the question: Do the benefits of applying machine learning for nature conservation outweigh the ecological and climate footprint? A new study led by CMCC looks at ML’s role in terrestrial ecology, analyzing 326 studies and concluding that algorithms hold great promise for delivering more accurate, scalable, and timely data, advancing real-time monitoring and near-instantaneous predictions for applications such as in seasonal forecasting.
From predicting where species live and understanding ecological dynamics, to identifying species traits and behaviors, improving conservation planning, and detecting environmental changes more quickly and accurately, machine learning (ML) can help generate faster responses to global challenges, including climate change, biodiversity loss, and land degradation.
“As ML algorithms can be quite hard to navigate, in this study we try demystifying the ‘AI jungle’ for ecologists, offering a clear overview of the different ways computers can learn from data, with a focus on algorithm families, strengths, limitations, and real-world applications in terrestrial ecology,” says CMCC researcher Cristina Cipriano – lead author of the review titled “Algorithms going wild – A review of machine learning techniques for terrestrial ecology”.
The core message of the study is that while ML offers powerful new possibilities for ecology in general, its full potential is still far from being reached due to several challenges, including the complexity and difficulty in interpretation of many models, the need for large amounts of high-quality data, and the tendency towards reinforcing biases (for example, by over-representing well-studied regions or species). Additionally, advanced ML requires significant computing power, which comes with environmental costs.
“These technical and environmental barriers can limit access to ML for many ecologists and decision-makers, particularly in under-resourced regions, and raise a variety of ethical concerns about fairness and transparency,” says Cipriano. “There’s also a clear paradox. ML is being used to help protect ecosystems and address climate change, yet its use can have significant environmental and climate costs through activities that contribute to emissions and resource depletion. This raises a critical question: Do the benefits of applying ML for nature conservation outweigh its ecological and climate footprint? The answer depends on how, where, and why ML is used with the review stressing the need for thoughtful, purpose-driven applications that maximize ecological value while minimizing environmental harm.”
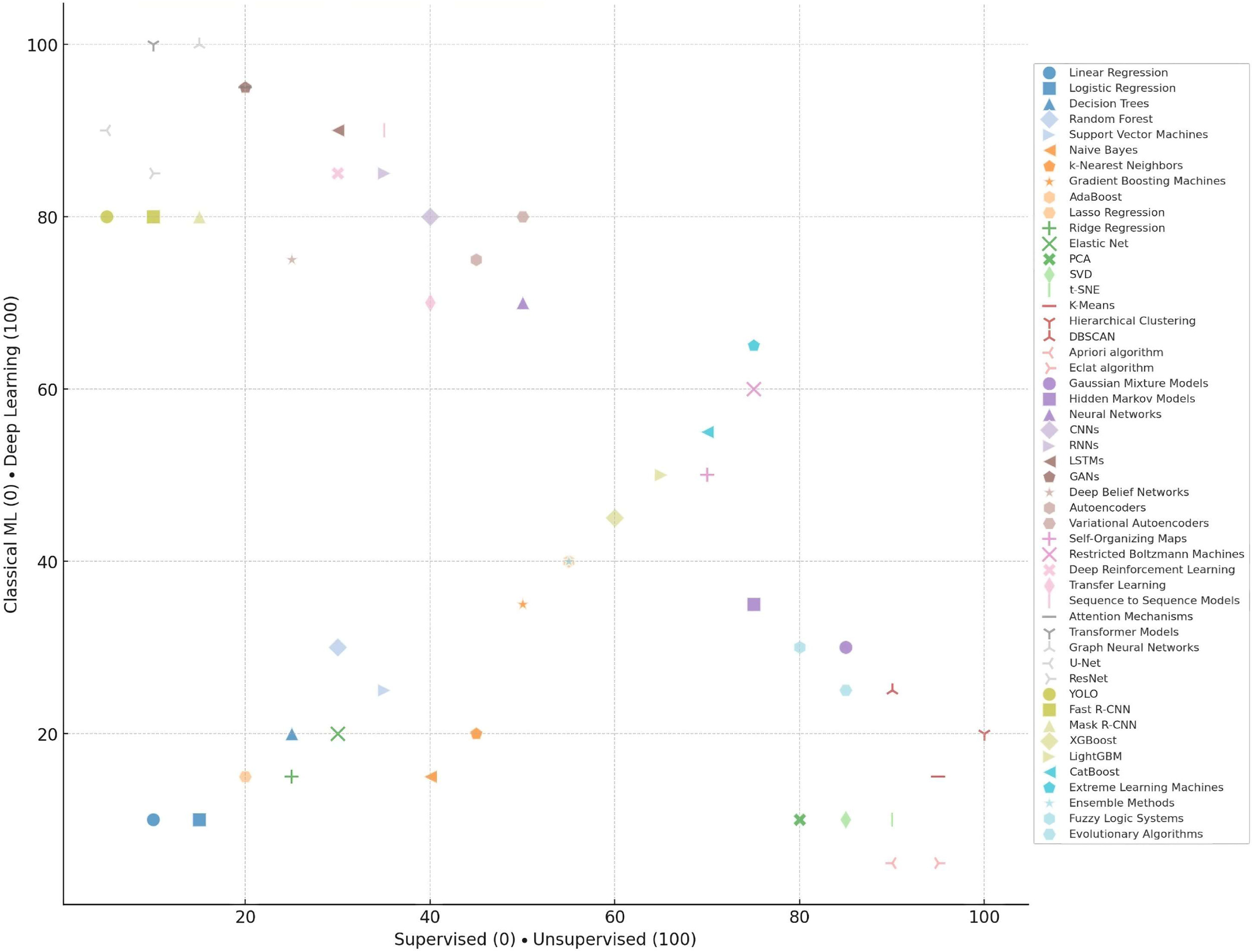
A scatter plot that visually maps different ML algorithms based on two key criteria: algorithm complexity (ranging from simpler, classical models to more complex deep learning architectures) and interpretability (how supervised or unsupervised the model is, as supervised models tend to be more interpretable because their structure is guided, while unsupervised models often lack this transparency, making their results harder to explain). Source: Cipriano et al., 2025
The figure above provides a quick, intuitive understanding of where each algorithm stands, whether it’s easier to interpret but less flexible, or highly powerful but harder to understand. For example, decision trees are low in complexity and highly interpretable, while deep neural networks are more complex and less transparent. Hybrid models fall somewhere in between. The placement of each algorithm in the plot is based on well-established literature, real-world usage patterns, and expert judgement, ensuring both rigor and usability. While the paper is qualitative in its nature, this figure stands out as a practical guide to support better-informed choices in ecological modeling, especially for those who may not have a background in computer science.
Ecological and ML communities are already taking steps to improve ecological data quality and standardization, making datasets more complete, diverse, and usable for ML. Researchers are exploring transfer learning and data augmentation to make better use of limited data, especially for rare species or underrepresented regions.
There’s also growing interest in explainable AI, tools that make model predictions easier to understand, and in integrating causal inference to uncover actual ecological processes. Importantly, more attention is being given to ethical design, including reducing the environmental footprint of models, identifying and addressing algorithmic biases, and promoting open, inclusive technologies that are accessible across different contexts. These developments show that the field is not only advancing technically, but also starting to respond to broader ecological and societal responsibilities.
“Our study also calls for stronger collaboration between ecologists and computer scientists, the development of open and user-friendly tools, and clearer communication about how models work and what their results mean,” says Cipriano. “This is crucial because ML could become a central tool in how we understand and care for nature, but only if it is made accessible, interpretable, fair, grounded in real-world ecological knowledge, and if its environmental and ethical implications are actively addressed.”
For more information:
Cristina Cipriano, Sergio Noce, Simone Mereu, Monia Santini, Algorithms going wild – A review of machine learning techniques for terrestrial ecology, Ecological Modelling, Volume 506, 2025, 111164, ISSN 0304-3800, https://doi.org/10.1016/j.ecolmodel.2025.111164.