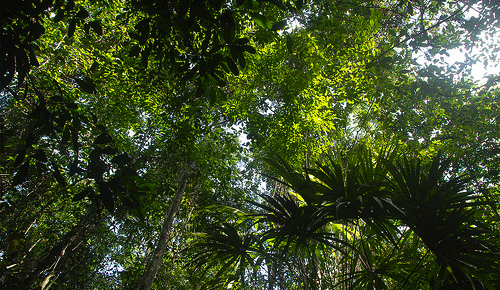
The estimation of above ground biomass in forests is critical for carbon cycle modeling and climate change mitigation programs. Small footprint lidar provides accurate biomass estimates, but its application in tropical forests has been limited, particularly in Africa. Hyperspectral data record canopy spectral information that is potentially related to forest biomass.
In a new study recently published on ISPRS Journal of Photogrammetry and Remote Sensing, a team of scientists (among them, CMCC researchers G. Vaglio Laurin and R. Valentini from IAFENT Division) tried to assess lidar ability to retrieve biomass in an African forest and the usefulness of including hyperspectral information.
Findings highlighted that the integration of hyperspectral bands improved the model based on lidar alone, this encouraging result call for additional research to clarify the possible role of hyperspectral data in tropical regions. Moreover, the combined use of lidar and hyperspectral data in tropical forest seem to improve biomass estimation.
The abstract of the paper:
The estimation of above ground biomass in forests is critical for carbon cycle modeling and climate change mitigation programs. Small footprint lidar provides accurate biomass estimates, but its application in tropical forests has been limited, particularly in Africa. Hyperspectral data record canopy spectral information that is potentially related to forest biomass. To assess lidar ability to retrieve biomass in an African forest and the usefulness of including hyperspectral information, we modeled biomass using small footprint lidar metrics as well as airborne hyperspectral bands and derived vegetation indexes. Partial Least Square Regression (PLSR) was adopted to cope with multiple inputs and multicollinearity issues; the Variable of Importance in the Projection was calculated to evaluate importance of individual predictors for biomass. Our findings showed that the integration of hyperspectral bands (R2 = 0.70) improved the model based on lidar alone (R2 = 0.64), this encouraging result call for additional research to clarify the possible role of hyperspectral data in tropical regions. Replacing the hyperspectral bands with vegetation indexes resulted in a smaller improvement (R2 = 0.67). Hyperspectral bands had limited predictive power (R2 = 0.36) when used alone. This analysis proves the efficiency of using PLSR with small-footprint lidar and high resolution hyperspectral data in tropical forests for biomass estimation. Results also suggest that high quality ground truth data is crucial for lidar-based AGB estimates in tropical African forests, especially if airborne lidar is used as an intermediate step of upscaling field-measured AGB to a larger area.
Read the integral version of the paper:
Vaglio Laurin G., Chen Q., Lindsell J., Coomes D. A. , Del Frate F., Guerriero L., Pirotti F., Valentini R.
Above ground biomass estimation in an African tropical forest with lidar and hyperspectral data
2014, ISPRS Journal of Photogrammetry and Remote Sensing, Volume 89, Pages 49–58, DOI: 10.1016/j.isprsjprs.2014.01.001