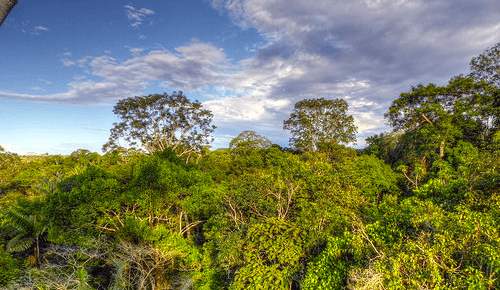
The spatial distribution of aboveground biomass and basal area are fundamental information in forestry. Moreover, the estimation of above ground biomass in forests is essential for carbon cycle modeling and climate change mitigation programs and strategies. A new study recently published on Remote Sensing (among the authors, CMCC researchers G. Vaglio Laurin and R. Valentini) tested metrics from full-waveform (FW) LiDAR (light detection and ranging) as predictors for forest basal area and aboveground biomass in a tropical moist forest in the south-western Ghana. The positive results in this study adds significant confirmation to other investigations that point to the success of LiDAR data towards monitoring forest resources from space-borne sensors.
The abstract of the paper:
We tested metrics from full-waveform (FW) LiDAR (light detection and ranging) as predictors for forest basal area (BA) and aboveground biomass (AGB), in a tropical moist forest. Three levels of metrics are tested: (i) peak-level, based on each return echo; (ii) pulse-level, based on the whole return signal from each emitted pulse; and (iii) plot-level, simulating a large footprint LiDAR dataset. Several of the tested metrics have significant correlation, with two predictors, found by stepwise regression, in particular: median distribution of the height above ground (nZmedian) and fifth percentile of total pulse return intensity (i_tot5th). The former contained the most information and explained 58% and 62% of the variance in AGB and BA values; stepwise regression left us with two and four predictors, respectively, explaining 65% and 79% of the variance. For BA, the predictors were standard deviation, median and fifth percentile of total return pulse intensity (i_totstdDev, i_totmedian and i_tot5th) and nZmedian, whereas for AGB, only the last two were used. The plot-based metric showed that the median height of echo count (HOMTC) performs best, with very similar results as nZmedian, as expected. Cross-validation allowed the analysis of residuals and model robustness. We discuss our results considering our specific case scenario of a complex forest structure with a high degree of variability in terms of biomass.
Read the integral version of the paper:
Pirotti F., Vaglio Laurin G., Vettore A. Masiero A. and Valentini R.
Small Footprint Full-Waveform Metrics Contribution to the Prediction of Biomass in Tropical Forests
2014, Remote Sensing, 6(10), 9576-9599; doi:10.3390/rs6109576