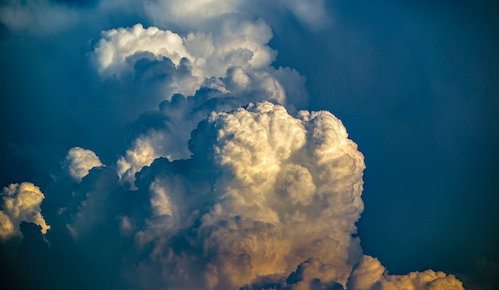
Machine learning is well suited for problems which have a complex structure that we don’t understand well, but about which we have huge amounts of information. For global weather and climate models, cumulus cloud systems present such a problem. They produce most of the world’s rainfall.
They involve interactions between air motions, clouds, liquid and ice precipitation, all poorly resolved by a typical model horizontal grid spacing of 10-100 km, which we represent with uncertain ‘subgrid parameterizations’.
We can simulate these interactions well with a much finer model grid of 1-5 km that explicitly simulate individual cumulus clouds, but this is too computationally expensive for normal use in a climate model.
On November 13, 2018, Prof. Christopher S. Bretherton (Departments of Atmospheric Science and Applied Mathematics, University of Washington, USA) explored this issue during the CMCC webinar “Machine learning and cloud process parameterization for weather and climate models”.
Watch the video:
The webinar explores machine learning for development of new parameterizations of cloud processes for climate models, based on large amounts of training data from 1-5 km models. The goal is that such parameterizations be easier to develop, more consistent with observations, and more efficient than what we use now.